Checking for non-preferred file/folder path names (may take a long time depending on the number of files/folders) ...
This resource contains some files/folders that have non-preferred characters in their name. Show non-conforming files/folders.
This resource contains content types with files that need to be updated to match with metadata changes. Show content type files that need updating.
Data for Measuring porous media velocity fields and grain bed architecture with a quantitative PLIF-based technique
Authors: |
|
|
---|---|---|
Owners: |
|
This resource does not have an owner who is an active HydroShare user. Contact CUAHSI (help@cuahsi.org) for information on this resource. |
Type: | Resource | |
Storage: | The size of this resource is 4.7 GB | |
Created: | Mar 07, 2023 at 9:36 p.m. | |
Last updated: | Sep 25, 2023 at 12:57 p.m. | |
DOI: | 10.4211/hs.a79d513a08064ecd85f781bb9dfb642d | |
Citation: | See how to cite this resource | |
Content types: | File Set Content |
Sharing Status: | Published |
---|---|
Views: | 523 |
Downloads: | 15 |
+1 Votes: | Be the first one to this. |
Comments: | No comments (yet) |
Abstract
Porous media flows are common in both natural and anthropogenic systems. Mapping these flows in a laboratory setting is challenging and often requires non-intrusive measurement techniques, such as particle image velocimetry (PIV) coupled with refractive index matching (RIM). RIM-coupled PIV allows the mapping of velocity fields around transparent solids by analyzing the movement of neutrally buoyant micron-sized seeding particles. The use of this technique in a porous medium can be problematic because seeding particles adhere to grains, which causes the grain bed to lose transparency and can obstruct pore flows. Another non-intrusive optical technique, planar laser-induced fluorescence (PLIF), can be paired with RIM and does not have this limitation because fluorescent dye is used instead of particles, but it has been chiefly used for qualitative flow visualization. Here, we propose a quantitative PLIF-based methodology to map both porous media flow fields and porous media architecture. Velocity fields are obtained by tracking the advection-dominated movement of the fluorescent dye plume front within a porous medium. We also propose an automatic tracking algorithm that quantifies 2D velocity components as the plume moves through space in both an Eulerian and a Lagrangian framework. We apply this algorithm to three data sets: a synthetic data set and two laboratory experiments. Performance of this algorithm is reported by the mean (bias error, B) and standard deviation (random error, SD) of the residuals between its results and the reference data. For the synthetic data, the algorithm produces maximum errors of B & SD = 32% & 23% in the Eulerian framework, respectively, and B & SD = −0.04% & 3.9% in the Lagrangian framework. The small-scale laboratory experimental data requires the Eulerian framework and produce errors of B & SD = −0.5% & 33%. The Lagrangian framework is used on the large-scale laboratory experimental data and produces errors of B & SD = 5% & 44%. Mapping the porous media architecture shows negligible error for reconstructing calibration grains of known dimensions. Article DOI: 10.1088/1361-6501/acfb2b
Subject Keywords
Content
Additional Metadata
Name | Value |
---|---|
Paper DOI | https://doi.org/10.1088/1361-6501/acfb2b |
Image Names | All Images are titled "B" followed by their respective frame number of the data set. Increasing frame numbers indicates frames taken later in time. |
How to Cite
This resource is shared under the Creative Commons Attribution CC BY.
http://creativecommons.org/licenses/by/4.0/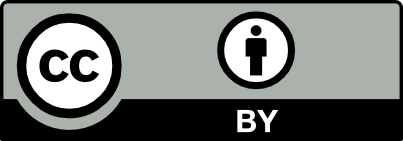
Comments
There are currently no comments
New Comment