Checking for non-preferred file/folder path names (may take a long time depending on the number of files/folders) ...
This resource contains some files/folders that have non-preferred characters in their name. Show non-conforming files/folders.
This resource contains content types with files that need to be updated to match with metadata changes. Show content type files that need updating.
Authors: |
|
|
---|---|---|
Owners: |
|
This resource does not have an owner who is an active HydroShare user. Contact CUAHSI (help@cuahsi.org) for information on this resource. |
Type: | Resource | |
Storage: | The size of this resource is 64.7 MB | |
Created: | Apr 11, 2024 at 8:34 a.m. | |
Last updated: | Apr 11, 2024 at 2:55 p.m. | |
Citation: | See how to cite this resource |
Sharing Status: | Public |
---|---|
Views: | 26 |
Downloads: | 0 |
+1 Votes: | Be the first one to this. |
Comments: | No comments (yet) |
Abstract
This folder contains output files from Spieler & Schütze (2024), Investigating the Model Hypothesis Space: Benchmarking Automatic Model Structure Identification with a Large Model Ensemble, Water Resources Research (in review)
Output files three modelling experiments conducted in the paper are presented.
The data for the Automatic Model Structure Identification (AMSI) experiment contains:
- 1 table of all 100 AMSI models for each of the 12 catchments. Included is the KGE in calibration and validation, the rank in calibration and validation as well as the identified structural choices for every model
- 1 table of all 100 AMSI models for each of the 12 signatures. Included are 79 hydrological signatures for each of the 100 models. They are ranked after their calibration performance.
The data for the Brute-Force-Modelling (BFM) experiment contains:
- 1 table of all 7488 BFM models for each of the 12 catchments. Included is the KGE in calibration and validation, the rank in calibration and validation as well as the corresponding structural choices of every model
- 1 table of all 7488 BFM models for each of the 12 signatures. Included are 79 hydrological signatures for each of the models. They are ranked after their calibration performance.
The data for the MARRMoT (MRMT) experiment contains:
- 1 table of all 45 MRMT models for each of the 12 catchments. Included is the KGE in calibration and validation, the rank in calibration and validation as well as the corresponding model ID of every model
- 1 table of all 45 MRMT models for each of the 12 signatures. Included are 79 hydrological signatures for each of the models. They are ranked after their calibration performance.
Study abstract:
Selecting an appropriate model for a catchment is challenging, and choosing an inappropriate model can yield unreliable results. The Automatic Model Structure Identification (AMSI) method simultaneously calibrates model structural choices and model parameters, which reduces the workload of comparing different models. We benchmark AMSI’s capabilities in two ways, using 12 hydro-climatically diverse MOPEX catchments. First, we calibrate parameter values for 7488 different model structures and test AMSI’s ability to find the best-performing models in this set. Second, we compare the performance of these 7488 models and AMSI’s selection to the performance of 45 commonly used, structurally more diverse, conceptual models. In both cases, we quantify model accuracy (through the Kling-Gupta Efficiency) and model adequacy (through various hydrologic signatures). AMSI effectively identifies high-accuracy models among the 7488 options, with KGE scores comparable to the best among the 45 models. However, model adequacy remains poor for the accurate models, regardless of the selection method. In nine of the tested catchments, none of the most accurate models replicate observed signatures with less than 50% errors; in the remaining three catchments, only a handful of models do so. This paper thus provides strong empirical evidence that relying on aggregated efficiency metrics is unlikely to result in hydrologically adequate models, no matter how the models themselves are selected. Nevertheless, AMSI has been shown to effectively search the model hypothesis space it was given. Combined with an improved calibration approach it can therefore offer new ways to address the challenges of model structure selection.
Subject Keywords
Coverage
Temporal
Start Date: | |
---|---|
End Date: |
Content
How to Cite
This resource is shared under the Creative Commons Attribution CC BY.
http://creativecommons.org/licenses/by/4.0/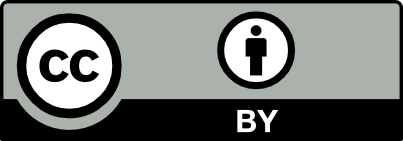
Comments
There are currently no comments
New Comment