Checking for non-preferred file/folder path names (may take a long time depending on the number of files/folders) ...
This resource contains some files/folders that have non-preferred characters in their name. Show non-conforming files/folders.
This resource contains content types with files that need to be updated to match with metadata changes. Show content type files that need updating.
Authors: |
|
|
---|---|---|
Owners: |
|
This resource does not have an owner who is an active HydroShare user. Contact CUAHSI (help@cuahsi.org) for information on this resource. |
Type: | Resource | |
Storage: | The size of this resource is 56.2 MB | |
Created: | Apr 30, 2022 at 12:58 a.m. | |
Last updated: | May 10, 2022 at 4:54 a.m. | |
DOI: | 10.4211/hs.08e1b18aa9f240758dd13d9ac875621f | |
Citation: | See how to cite this resource |
Sharing Status: | Published |
---|---|
Views: | 567 |
Downloads: | 26 |
+1 Votes: | Be the first one to this. |
Comments: | No comments (yet) |
Abstract
Quantifying continental-scale river discharge is essential for understanding the terrestrial water cycle, which is susceptible to errors due to lack of observations or limitations in hydrodynamic modelling. Data assimilation (DA) methods are increasingly utilized to estimate river discharge combined with the emerging amount of river-related remote sensing data (e.g., water surface elevation, water surface slope, river width, flood extent, etc.). However, direct comparison of simulated water surface elevation (WSE) with the satellite altimetry data remains still challenging (i.e., large bias between simulations and observation, uncertainty in parameters, etc.) and can introduce large errors when assimilating satellite observations to hydrodynamic models. We performed several experiments, namely, direct, anomaly, and normalized value assimilations, to investigate the capability of DA to improve the river discharge with the current limitations of hydrodynamic modelling. The hydrological data assimilation was performed using a physically-based empirical localization method in the Amazon Basin. We used satellite altimetry data from ENVISAT, Jason 1 and Jason 2 for this study. The direct DA was used as the baseline of the assimilations, but it was subjected to errors due to the biases in the simulated WSE. As an alternative to direct DA, we used anomaly DA to overcome the errors due to the biases in the simulated WSE. In addition, we found that the modelled WSE distribution and the observed distribution differed considerably (i.e., amplitude differences, seasonal flow variations, distribution skewness due to limitations of hydrodynamic models, etc.). Therefore, a normalized value DA was performed to realize better discharge estimation. River discharge improved in 24%, 38%, and 62% of the stream gauges in the direct, anomaly, and normalized value assimilations compared to simulations without DA. The normalized value assimilation performed better in estimating river discharge given the current limitations of hydrodynamic models. Most of the gauges within the river reaches with satellite observations accurately estimated the river discharge with Nash-Sutcliffe Efficiency (NSE) > 0.6. The amplitudes of WSE were improved in the normalized DA experiment. Furthermore, in the Amazon Basin, normalized assimilation (median NSE=0.47) can improve river discharge estimation over the open-loop simulation with global hydrodynamic modeling (median NSE=0.13). River discharge estimation by direct DA methods can be improved by 7% of NSE by calibrating river bathymetry. Moreover, the direct DA approach outperforms the other DA methods when the runoff is considerably (50%) biased. The uncertainties in hydrodynamic modelling (i.e., river bottom elevation, river width, simplified floodplain dynamics, rectangular cross-section assumption, etc.) should be improved for better estimation of river discharge by assimilating satellite altimetry. This study will contribute to developing a global river discharge reanalysis product that is consistent spatially and temporally.
Subject Keywords
Coverage
Spatial
Content
Related Resources
The content of this resource was created by a related App or software program | DOI: 10.5281/zenodo.6506861 |
How to Cite
This resource is shared under the Creative Commons Attribution CC BY.
http://creativecommons.org/licenses/by/4.0/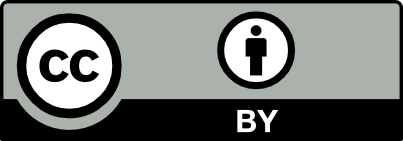
Comments
There are currently no comments
New Comment