Checking for non-preferred file/folder path names (may take a long time depending on the number of files/folders) ...
This resource contains some files/folders that have non-preferred characters in their name. Show non-conforming files/folders.
This resource contains content types with files that need to be updated to match with metadata changes. Show content type files that need updating.
Authors: |
|
|
---|---|---|
Owners: |
|
This resource does not have an owner who is an active HydroShare user. Contact CUAHSI (help@cuahsi.org) for information on this resource. |
Type: | Resource | |
Storage: | The size of this resource is 40.6 MB | |
Created: | Mar 13, 2024 at 6:41 p.m. | |
Last updated: | Mar 14, 2024 at 5:03 p.m. | |
Citation: | See how to cite this resource |
Sharing Status: | Public |
---|---|
Views: | 41 |
Downloads: | 3 |
+1 Votes: | Be the first one to this. |
Comments: | No comments (yet) |
Abstract
Direct interpretation of complex and heterogeneous geological systems in terms of facies from individual tomograms remains a significant challenge because of noise in the measured data and non-uniqueness in geophysical inversion. We introduce a machine learning-based approach for hydrogeophysical image reconstruction using the Expectation-Maximization algorithm for joint classification of distinct hydrofacies from two or more independently inverted geophysical data sets.
To understand the impact of noise on facies discrimination, we design two synthetic models of hydrofacies with varying levels of complexity and heterogeneity. This synthetic study allows us to compare the classified image with the benchmark model for different noise scenarios. With field data in the Laramie range, WY, USA, we explore the effects of regularization on the hydrofacies classification.
The dataset consists of electrical resistivity and seismic velocities in both the field case and the synthetic case.
Subject Keywords
Coverage
Spatial
Temporal
Start Date: | |
---|---|
End Date: |
Content
How to Cite
This resource is shared under the Creative Commons Attribution CC BY.
http://creativecommons.org/licenses/by/4.0/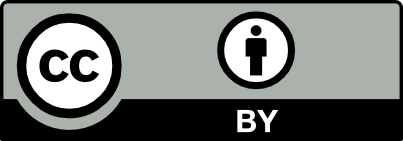
Comments
There are currently no comments
New Comment